Bridging the performance gap in data infrastructure for AI
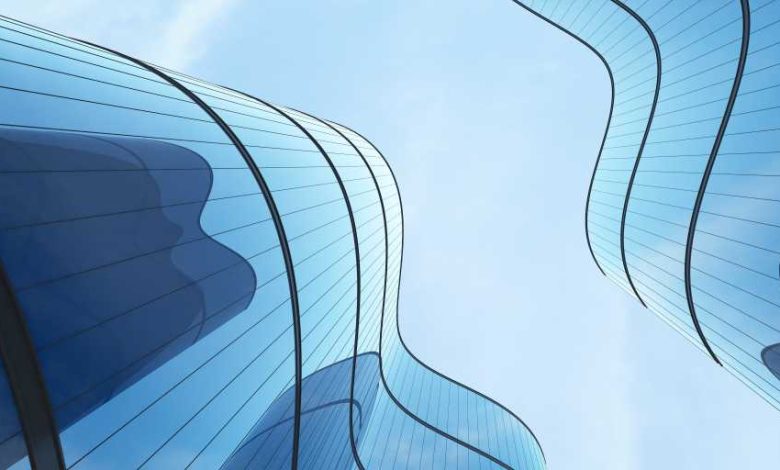
One instance, the VAST Information Platform, provides unified storage, database, and data-driven perform engine companies constructed for AI, enabling seamless entry and retrieval of knowledge important for AI mannequin growth and coaching. With enterprise-grade safety and compliance options, the platform can seize, catalog, refine, enrich, and protect information by real-time deep information evaluation and studying to make sure optimum useful resource utilization for quicker processing, maximizing the effectivity and pace of AI workflows throughout all levels of a knowledge pipeline.
Hybrid and multicloud methods
It may be tempting to select a single hyperscaler and use the cloud-based structure they supply, successfully “throwing cash on the downside.” But, to attain the extent of adaptability and efficiency required to construct an AI program and develop it, many organizations are selecting to embrace hybrid and multicloud methods. By leveraging a mixture of on-premises, non-public cloud, and public cloud sources, companies can optimize their infrastructure to satisfy particular efficiency and value necessities, whereas garnering the flexibleness required to ship worth from information as quick because the market calls for it. This strategy ensures that delicate information may be securely processed on-premises whereas making the most of the scalability and superior companies provided by public cloud suppliers for AI workloads, thus sustaining excessive compute efficiency and environment friendly information processing.
Embracing edge computing
As AI functions more and more demand real-time processing and low-latency responses, incorporating edge computing into the information structure is turning into important. By processing information nearer to the supply, edge computing reduces latency and bandwidth utilization, enabling quicker decision-making and improved person experiences. That is notably related for IoT and different functions the place fast insights are essential, guaranteeing that the efficiency of the AI pipeline stays excessive even in distributed environments.